Advancing Fundamental Understanding of Retention Interactions in Supercritical Fluid Chromatography Using Artificial Neural Networks: Polar Stationary Phases with -OH Moieties
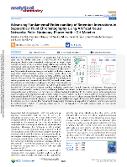
Autor
Gazárková, Taťána
Datum vydání
2024Publikováno v
Analytical ChemistryRočník / Číslo vydání
96 (31)ISBN / ISSN
ISSN: 0003-2700ISBN / ISSN
eISSN: 1520-6882Metadata
Zobrazit celý záznamTato publikace má vydavatelskou verzi s DOI 10.1021/acs.analchem.4c01811
Abstrakt
The retention behavior in supercritical fluid chromatography and its stability over time are still unsatisfactorily explained phenomena despite many important contributions in recent years, especially focusing on linear solvation energy relationship modeling. We studied polar stationary phases with predominant -OH functionalities, i.e., silica, hybrid silica, and diol columns, and their retention behavior over time. We correlated molecular descriptors of analytes with their retention using three organic modifiers of the CO2-based mobile phase. The differences in retention behavior caused by using additives, namely, 10 mmol/L NH3 and 2% H2O in methanol, were described in correlation to analyte properties and compared with the CO2/methanol mobile phase. The structure of >100 molecules included in this study was optimized by semiempirical AM1 quantum mechanical calculations and subsequently described by 226 molecular descriptors including topological, constitutional, hybrid, electronic, and geometric descriptors. An artificial neural networks simulator with deep learning toolbox was trained on this extensive set of experimental data and subsequently used to determine key molecular descriptors affecting the retention by the highest extent. After comprehensive statistical analysis of the experimental data collected during one year of column use, the retention on different stationary phases was fundamentally described. The changes in the retention behavior during one year of column use were described and their explanation with a proposed interpretation of changes on the stationary phase surface was suggested. The effect of the regeneration procedure on the retention was also evaluated. This fundamental understanding of interactions responsible for retention in SFC can be used for the evidence-based selection of stationary phases suitable for the separation of particular analytes based on their specific physicochemical properties.
Klíčová slova
supercritical fluid chromatography, artificial neural networks, stationary phases
Trvalý odkaz
https://hdl.handle.net/20.500.14178/2571Licence
Licence pro užití plného textu výsledku: Creative Commons Uveďte původ 4.0 International