Learnability of state spaces of physical systems is undecidable
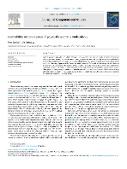
Datum vydání
2024Publikováno v
Journal of Computational ScienceRočník / Číslo vydání
83 (December 2024)ISBN / ISSN
ISSN: 1877-7503ISBN / ISSN
eISSN: 1877-7511Metadata
Zobrazit celý záznamKolekce
Tato publikace má vydavatelskou verzi s DOI 10.1016/j.jocs.2024.102452
Abstrakt
Despite an increasing role of machine learning in science, there is a lack of results on limits of empirical exploration aided by machine learning. In this paper, we construct one such limit by proving undecidability of learnability of state spaces of physical systems. We characterize state spaces as binary hypothesis classes of the computable Probably Approximately Correct learning framework. This leads to identifying the first limit for learnability of state spaces in the agnostic setting. Further, using the fact that finiteness of the combinatorial dimension of hypothesis classes is undecidable, we derive undecidability for learnability of state spaces as well. Throughout the paper, we try to connect our formal results with modern neural networks. This allows us to bring the limits close to the current practice and make a first step in connecting scientific exploration aided by machine learning with results from learning theory.
Klíčová slova
undecidability, machine learning, probably approximately correct learning, scientific exploration, deep neural networks
Trvalý odkaz
https://hdl.handle.net/20.500.14178/2678Licence
Licence pro užití plného textu výsledku: Creative Commons Uveďte původ 4.0 International